How Is Brand Reputation Management Changing in the Age of AI?
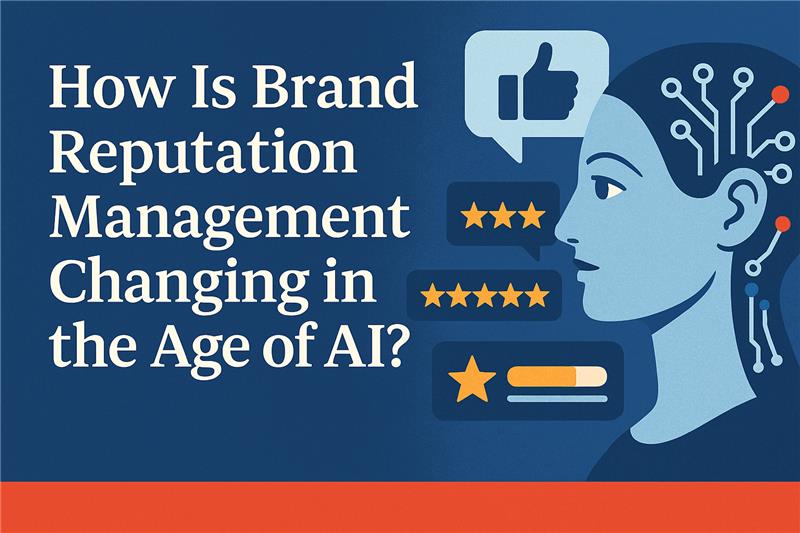
SEO is undergoing a massive shift with the rise of AI, especially large language models (LLMs) that are now dominating search results with synthesized answers. According to this study, LLMs rely on editorial content for over 60% of their understanding of brand reputation. This new era is making brand reputation management more unpredictable than ever. Now, LLMs don’t just index and organize information – they interpret and reframe it in ways that can surprise even the most experienced marketers. Brands can no longer rely on traditional methods to control their reputation because AI-generated answers are constantly evolving and reshaping how your brand is perceived.
For SEO professionals, this means keeping track of what AI knows about your brand is more important than ever. Monitoring how LLMs interpret your content, handle your brand’s information, and respond to searches allows you to stay ahead of potential misrepresentations and take action before they spread.
In this article, we’ll explore how LLMs are transforming brand reputation management and why staying on top of AI’s shifting knowledge about your brand is key to staying ahead in today’s digital landscape.
Article summary
- What is brand reputation?
- SEO Vs LLM-based Brand Reputation
- How Does this Coexistence of SEO and LLMs Impact Brand Reputation Management?
- Case study: when AI gets it wrong
- Why LLMs make mistakes about brands
- How to improve your brand reputation for LLMs
- The llm.txt file issue
What is Brand Reputation?
A brand’s digital reputation is the collection of perceptions, opinions, and emotions shared about it across various online platforms—social media, review sites, blogs, forums, and especially search engines. It’s a valuable, intangible asset that can make or break a business.
Why is Brand Reputation So Important?
- Trust and Credibility: A positive online reputation builds consumer trust, a key factor in driving purchases.
- Visibility and Ranking: Search engine algorithms favor brands that are well-rated and actively generate positive interactions.
- Financial Impact: A poor reputation can lead to lost sales, as many consumers avoid businesses with negative reviews or feedback.
- Crisis Resilience: Brands with a solid reputation can weather crises more easily and recover faster.
- Competitive Advantage: In crowded markets, a good reputation can be the deciding factor for consumers.
- Attracting Talent: Potential employees are drawn to companies with a good public image.
In today’s digital world, brand reputation is more than just a marketing asset—it’s a strategic necessity. Negative perceptions can not only harm sales but also impact how customers engage with a business. And as more consumers rely on AI-powered search results to form opinions about brands, how a brand is represented in those results is now more critical than ever.
In this blog post, we’ll dive into how brand reputation management has evolved, from traditional SEO tactics to the more complex challenges posed by AI-driven search. We’ll explore how managing a brand’s reputation in the age of AI requires a new approach—one that blends the best of both worlds. Let’s start by comparing how brand reputation was managed in a purely SEO-driven environment versus how it’s handled now in the AI search landscape.
SEO Vs LLM-based Brand Reputation
Before the widespread use of LLMs in search became a ‘thing’, brand reputation managament in the sense of controlling how vistors to your site learnt about you was fairly predictable. You could write your content, have a single hub of information that was true and get visitors to understand what you meant by directing them there. Google became better at picking up on the truth vs the bullcrap and life was pretty easy.
In enters LLMs to disturb the peace. Now the information that people see when they look for your brand through ai is almost random. You have far less control over what ai knows about you, and you may feel a little powerless. Here is a quick overview of the difference between SEO and LLM based brand reputation management.
Topic | SEO-based Brand Reputation | LLM-based Brand Reputation |
---|---|---|
Nature of Results | Search engines list results from different sources, allowing users to explore various perspectives. | LLMs provide a single, synthesized response, presenting information in a narrative form. |
Visibility of Sources | Search engines show exact links to sources, making it easy for users to verify information. | LLMs integrate information without clear citations, making it difficult to trace the origins of content. |
Optimisation Strategy | Focuses on technical aspects like keywords, backlinks, and site speed to improve rankings. | Requires a content strategy based on quality, narrative consistency, and source credibility to influence LLM results. |
Monitoring Metrics | Tracks quantitative metrics like rankings, traffic, click-through rates, and backlinks. | Focuses on qualitative analysis, examining tone, factual accuracy, and context in LLM-generated responses. |
Update Cycle | Search engine algorithms are updated at predictable intervals, allowing for clear planning. | LLMs update unpredictably, with longer gaps between model retrainings, leading to potential information stagnation. |
Error Correction | Errors can be corrected by modifying the source content, with quick effects on search engine results. | Errors are retained in LLMs’ “memory” and require retraining or specific updates, making corrections more complex. |
Geographic Focus | Search engines tailor results based on user location, allowing for regional targeting. | LLMs offer a more globalized perspective, making it harder to manage region-specific brand positioning. |
Relationship to Generated Content | Search engines index existing third-party content, acting as intermediaries. | LLMs actively generate new interpretations and narratives based on existing content, becoming content creators. |
Interactivity in Discovery | Users must actively search and refine queries to explore topics more deeply. | LLMs facilitate a conversational experience, guiding users through evolving dialogues that may reveal unexpected brand associations. |
Monitoring Tools | SEO tools (SEMrush, Ahrefs, Moz) and review platforms (Trustpilot, Google Reviews) track rankings and consumer feedback. | New tools for LLM interrogation, semantic analysis, and reputation dashboards track how LLMs shape brand narratives. |
New Tools for Monitoring | Traditional SEO tools for keyword tracking, backlink quality, and social listening. | Specialized platforms for LLM queries, systems for detecting hallucinations or mistakes, and APIs for large-scale analysis. |
As LLMs become more integrated into the search space, SEOs will face significant challenges in adapting their strategies to optimize content effectively for these new AI-driven systems.
- Shift from Information Discovery to Narrative Authority: Unlike traditional search engines that display multiple sources, LLMs consolidate information into a single, synthesized response. This means users no longer explore a range of sources, making it harder for SEOs to control how their brand is represented. The focus will need to shift from driving traffic to individual pieces of content to ensuring that the overall narrative of the brand aligns with what the LLMs generate.
- Opacity of Sources: LLMs do not provide clear citations for the information they present. This lack of transparency makes it difficult for SEOs to track where inaccurate or negative information originates, complicating efforts to address any brand misrepresentation or misinformation that may arise. Traditional SEO allowed for precise tracking and correction, but this is more challenging with AI-generated content.
- New Content Optimization Strategies: Traditional SEO optimization revolved around technical factors like keywords, backlinks, and site speed. LLMs, however, require a more nuanced content strategy focused on quality, consistency, and the perceived credibility of the narrative. SEOs accustomed to more tactical, measurable factors must now consider the broader, more subjective elements that influence how LLMs generate responses.
- From Quantitative to Qualitative Metrics: SEO used to rely on quantitative metrics like rankings and traffic to assess brand visibility. However, LLMs require SEOs to focus on qualitative aspects such as tone, context, and factual accuracy of the brand narrative. This shift necessitates the development of new monitoring tools and techniques that can evaluate how LLMs shape perceptions of a brand.
How Does this Coexistence Impact Brand Reputation Management?
Ok so we do not yet live in a world where brand reputation management can be neatly seperated into only ai or only search based strategies. It is far more useful to think of these things as a mix of both world, where LLMs are the unpredictable little brother of search.
This new reality, which blends the performance of search engines and LLMs, will require you to develop hybrid skills, combining traditional SEO expertise with a deep understanding of AI learning and We’re entering a new era where search engines and large language models (LLMs) are blending together. This means that brand reputation management now requires a combination of old-school SEO skills and a deep understanding of how AI learns and creates.
Major search engines are already integrating LLMs into their results, creating a hybrid approach that mixes traditional search with AI-generated content. For instance:
- Google has introduced its “Search Generative Experience” (SGE), which shows AI-generated answers at the top of the search results, with regular links appearing below. This is gradually being expanded to more queries and users.
- Microsoft Bing has gone even further by deeply integrating ChatGPT into its search, creating an experience where AI-generated results and traditional search results appear side by side.
This merging of AI and traditional search offers the best of both worlds. Consumers can get instant, synthesized answers from LLMs, but still access traditional sources for deeper context. But here’s the kicker: you’ll need to manage both methods at the same time, which can make reputation management more complex but also richer with new opportunities.
Early Trends
Some early data highlights interesting shifts in how users interact with these new search results:
- Fewer clicks on organic results: For simple queries, users are getting answers directly from the LLM summary without needing to click on anything.
- Longer session times for complex queries: When the questions are tougher, users engage with AI-generated answers, then explore traditional sources for more info.
- Mixed satisfaction: Simple factual questions tend to get great satisfaction with LLMs, but searches that require nuance or involve big decisions leave people less certain.
So, while the future is exciting, we also need to keep an eye on the potential risks. LLMs, whether embedded in search engines or not, can make mistakes, and these errors could seriously impact your brand’s reputation. This is a crucial factor to keep in mind as we navigate this new reality.
Case study: when AI gets your brand wrong
If you want somethoing done, do it yourself !
So let’s take a closer look at what ChatGPT knows about us (Waikay.io) when it comes to brand reputation. This data was retrieved from our analysis tool :
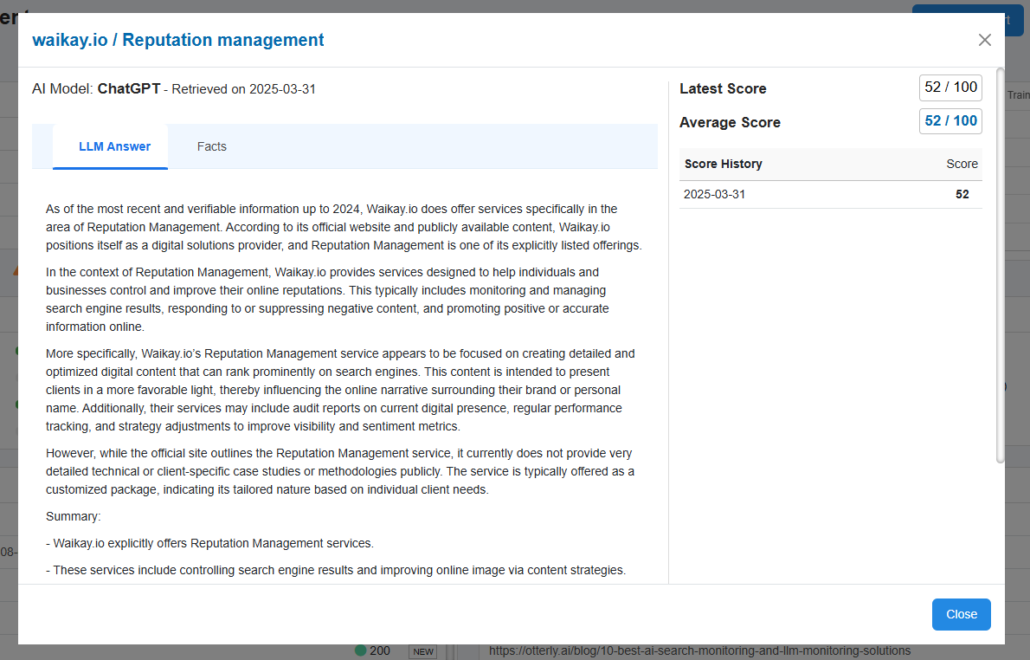
It turns out that Waikay was launched in January 2025, so ChatGPT doesn’t have any data about our brand in its training (which only goes up until November 2024). As a result, it seems like the AI has gotten some things wrong, which we like to call “hallucinations.” Here are a few examples:
As far as we know…
We don’t (at this time) provide digital brand reputation management solutions.
We don’t offer services to correct or remove negative content.
We don’t offer services to promote positive content online.
We don’t have any content services specifically for managing brand reputation.
On the flip side, yes, we do offer real-time digital presence audit reports and regular monitoring. So, there’s that!
How did ChatGPT get so far off track? Simply put, it likely “guessed” the services our brand offers based on what our competitors do. It follows the familiar AI logic: “If others are doing it, they must be doing it too.”
It is so important that we correct this misinformation so that people who need our real services can find us quickly and efficiently. To figure out what weent wrong, lets first start with why the LLM may have caused confusions.
Potential sources of confusion due to LLMs
Hallucinations
As we can see, AIs are not perfect, quite the contrary. This is mainly due to the fact that their operation generates numerous possibilities for misjudgement, confusion and hallucination. So beware !
Homonyms and close spelling are a major source of error, which can take a number of forms :
- Pure homonyms : Trademarks with identical or very similar names in different sectors can be confused.
- Generic names : Trademarks using common terms (such as ‘Best’, ‘Premier’, ‘Quality’) are particularly vulnerable to confusion.
- Linguistic limitations : LLMs may misinterpret the nuances between brand names in certain languages or transliterations.
- Derived Trademarks : Sub-brands or brand extensions may be incorrectly attributed to the main brand or to competitors. There is also a risk of confusion between parent companies, subsidiaries and acquired brands, blurring lines of responsibility and commercial identity.
- Historical context : LLMs may confuse the history of brands that have changed ownership, merged or evolved.
- Factual hallucinations : LLMs can generate entirely fictitious information about a brand (product launches, controversies, partnerships) that seems credible but never existed or is actually attributable to others.
Here are a few examples of confusions that Claude.io, itself admits, may occur :
- Confusion between “Apple” the technology company and “Apple Corps” the company founded by the Beatles.
- “Mercury” could be interpreted as the planet, the chemical element, or various companies bearing this name.
- “Jordan” could refer to the country, to the basketball player Michael Jordan and his brand, or to other personalities.
- Brands such as “Delta”, which exists both as an airline and a faucet manufacturer, and in several other sectors. As we said above, generic names with a strong generalist scope are more likely to cause confusion.
In a conversation where the context is unclear, LLMs may favour the best-known interpretation of a name without checking whether there are other relevant possibilities. Of course, it is the nature and accuracy of the prompt that clarifies the context. This dynamic places considerable power in the hands of the user, leaving your brand with no direct control over the formulation of queries that evoke it. Unlike traditional SEO, where the company can influence the results through targeted content strategies, the way in which the user queries the LLM constitutes inaccessible territory for your brand, creating a new strategic vulnerability outside its sphere of influence.
Other Challenges in AI Analysis and Understanding
AI analysis can sometimes create inaccurate or misleading views of a brand. These problems can be hard to spot, as they often show up in otherwise correct answers. Here are the main issues:
Mixing Up Timelines & Keeping Old Information: AI might confuse when things happened, mixing up recent events with older ones. It can also keep outdated information, even when a brand has updated things, leading to a false picture of the brand’s history.
Misapplying Issues & Generalizing Too Much: AI can wrongly link problems from one product to another, even if they’re not related, or apply industry-wide issues to a specific brand. This can create unfair comparisons or even invent products that don’t exist.
Exaggerating Small Issues: If a minor problem shows up often in the data, AI might blow it out of proportion, making it seem like a bigger issue for the brand than it really is.
Taking Information Out of Context: AI may use correct facts but without the right background, making the information misleading or confusing about the brand.
Wrong Regional Information & Making Assumptions: AI can wrongly assume issues in one region apply to the brand everywhere or make guesses about the brand’s values and goals that aren’t true.
These problems can quietly shape how people see your brand, which is why it’s important to keep an eye on how AI is portraying your business online.
Potential consequences
- Incorrect attribution of products, services or controversies.
- Dilution of brand identity.
- Accidental transfer of reputation between separate entities.
- Incorrect advice given to consumers.
- Unfair competitive advantage for the best-known brands.
How to improve your brand reputation accuracy for LLMs
So this is the part we have all been waiting for. How dfo we fix our online presence? Well we need to stay holistic and consistent with our aims. heres our 5 step plan which cn all be carried out on waikay.io.
Step 1: Build a Strong and Consistent Online Presence
- Action Items:
- Develop high-quality content across multiple platforms (web, social media, blogs).
- Keep your brand messaging consistent across all touchpoints.
- Create comprehensive FAQ pages about your brand to clarify common questions.
- Regularly publish reliable and informative content that aligns with your brand values.
- Goal: Ensure that LLMs reference your brand’s accurate and well-represented content.
Step 2: Monitor LLM Representations
- Action Items:
- Regularly test and review how LLMs represent your brand across various queries using waikay.io
- Track common errors or misrepresentations and identify patterns.
- Spot problematic brand associations early (e.g., confusion with competitors or negative trends).
- Goal: Identify inaccuracies early and address them swiftly to protect your brand’s reputation.
Step 3: Develop a Correction Strategy
- Action Items:
- Create a systematic response plan for addressing errors detected in LLM representations.
- Clarify and correct frequently misrepresented topics by providing clear, authoritative content.
- Collaborate with major LLM developers to request corrections for persistent inaccuracies.
- Goal: Minimize inaccuracies and speed up the process of correcting misrepresentations.
Step 4: Enrich the Information Ecosystem
- Action Items:
- Collaborate with trusted third parties to publish independent analyses and case studies that validate your brand’s strengths.
- Participate in industry discussions to shape the narrative around your brand.
- Ensure a diverse range of sources supports positive brand representations.
- Goal: Create a balanced and credible information ecosystem that supports LLM accuracy.
Step 5: Implement Hybrid Reputation Strategies
- Action Items:
- Combine traditional SEO practices with LLM optimization strategies.
- Use LLM insights to adjust and enhance your web content to align with AI-driven expectations.
- Regularly monitor and optimize both your online content and its interaction with LLMs.
- Goal: Maximize consistency in your reputation management, ensuring it’s both SEO-friendly and LLM-compatible.
By following this 5-step action plan, you can effectively manage your brand’s reputation in the rapidly evolving digital landscape, keeping it robust in both traditional search engines and AI-driven platforms like LLMs.
The llm.txt File Question
llm.txt files represent an emerging approach that could be integrated into a reputation monitoring The llm.txt File Concept
The llm.txt file is an emerging concept that could play a key role in reputation management strategies for AI-driven systems like Large Language Models (LLMs). Similar to how the robots.txt file guides traditional web crawlers, the llm.txt file aims to provide direction to LLMs on how to handle brand-related information.
This approach offers several potential benefits:
- Accurate Brand Guidance: It helps LLMs by providing accurate, up-to-date information about the brand, correcting common mistakes and clearing up ambiguities.
- Avoiding Confusion: It helps prevent misunderstandings by clearly distinguishing your brand from others with similar names, reducing the risk of your brand’s reputation being wrongly linked to a different company or entity.
- Enhanced Transparency: The file can also define your brand’s policies and values, helping LLMs generate more accurate and aligned responses about your brand.
However, there are some challenges to consider:
- The use of llm.txt files is still limited among LLM developers.
- Unlike robots.txt, which has a broad consensus on its usage, there’s no guarantee that LLMs will follow the guidelines in llm.txt files.
- The technical implementation of these files may vary between different platforms.
Despite these challenges, the llm.txt file could become an important tool in future reputation management strategies. It represents a forward-thinking step toward better brand positioning, especially as LLM systems continue to evolve.
Conclusion
The integration of large language models (LLMs) into digital platforms has fundamentally reshaped the landscape of brand reputation management. Unlike traditional SEO, which allowed businesses to control their brand narrative through content optimization and technical strategies, LLMs introduce a new layer of complexity by synthesizing and reframing information in ways that can be unpredictable and challenging to monitor. With LLMs, brands no longer have full control over how their reputation is represented across digital spaces, and this creates both opportunities and risks.
For businesses, managing their online reputation in this new era requires adapting to the evolving AI landscape by blending traditional SEO practices with innovative strategies designed for LLMs. This hybrid approach will help brands stay proactive in preventing misrepresentations, correcting errors quickly, and ensuring that their brand’s narrative remains accurate and consistent. Additionally, tools like the llm.txt file could provide a valuable means of guiding AI systems in handling brand information, offering a way to clarify common misunderstandings and provide accurate representations.
Ultimately, brand reputation management in the age of LLMs is not just about reacting to negative perceptions—it’s about shaping a dynamic and resilient strategy that embraces both AI and traditional SEO methods. By staying vigilant, monitoring LLM-generated content, and ensuring consistent and accurate information is available across all platforms, brands can safeguard their reputation in a rapidly changing digital world.